What We Do
Our Approach
- Develop
- Train and validate predictive and diagnostic algorithms using real-world data
- Plan
- Quantitative and mixed-methods exploration to identify optimal use cases and implementation strategies
- Test
- Implementation and prospective evaluation of algorithm-enabled interventions
- Scale
- Scaling algorithms for clinical impact
Latest Updates
Featured Project
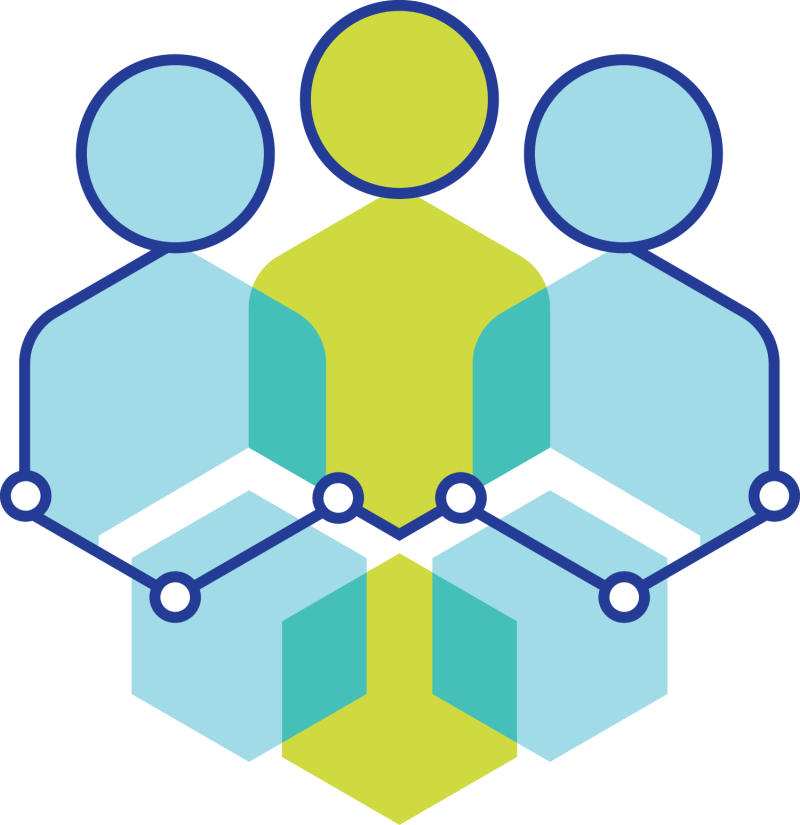
Behavioral Economics and analytics to improve PALliative care in advanced cancer
BE-a-PAL (Behavioral Economics and analytics to improve PALliative care in advanced cancer) was a pragmatic cluster-randomized trial across 15 community oncology practices. We utilizied algorithm-based default palliative care referrals embedded in the EHR with option for clinician opt-out. Rates of completed palliative care visits were 46.6% in the intervention arm compared to 11.3% in the control arm. In short, our intervention led to a 3-fold increase in specialty PC and decrease in end-of-life chemotherapy in a large community oncology network. Additional qualitative analysis among clinicians is ongoing.
Director Ravi Parikh presented results from BE-a-PAL at the 2024 ASCO Annual Meeting. PM Will Ferrell will be presenting study results at the upcoming 2024 AcademyHealth Annual Research Meeting.
HAC Blog
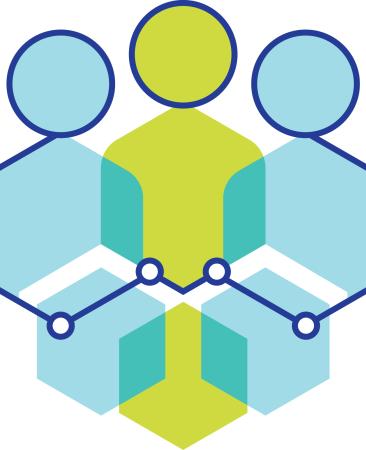
Staff Spotlight #3
Updates from HACLab for June 2024 including highlights from the 2024 ASCO Annual Meeting!
Author: Will Ferrell
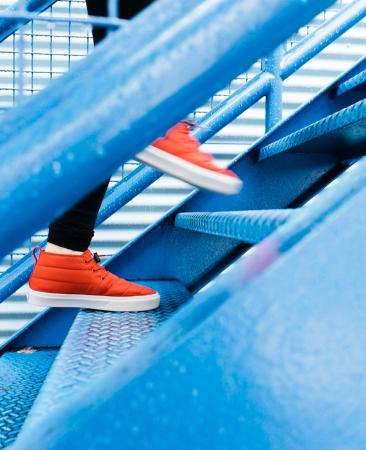
PGHD in the HACLab
This blog highlights patient-generated health data (PGHD) by reviewing a case study of one of our clinical trials: PROStep: A Feasibility Trial Using PROs and Step Data to Monitor Patients With Lung and GI Cancers
Author: Will Ferrell
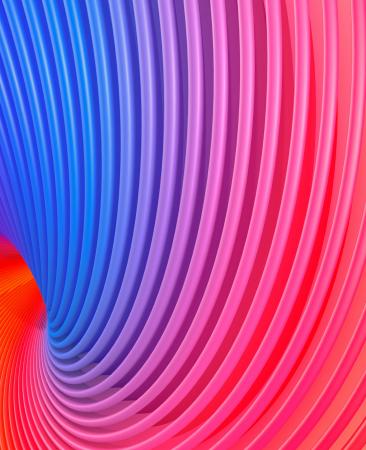
Statistical Concepts and Metrics of Algorithm Bias and Fairness
In the first entry of this series, we examined how algorithms can be biased on a variety of factors, including their underlying data, biased individuals creating models, or from statistical mischaracterizations from models themselves. In this entry, Caleb Hearn et al. explore how bias in algorithms is identified and quantified according to a few different statistical concepts and metrics.
Authors: Caleb Hearn, Sae-Hwan Park, Ravi Parikh
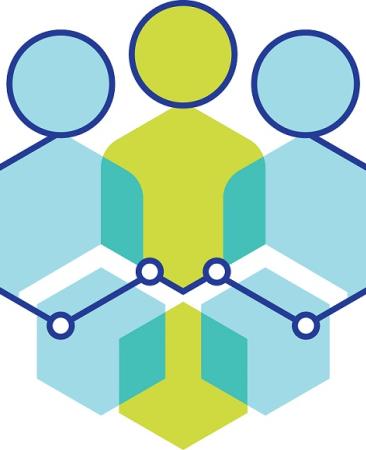
HACBlog: Staff Spotlight #2
Updates from the HACLab for October 2023
Author: Will Ferrell
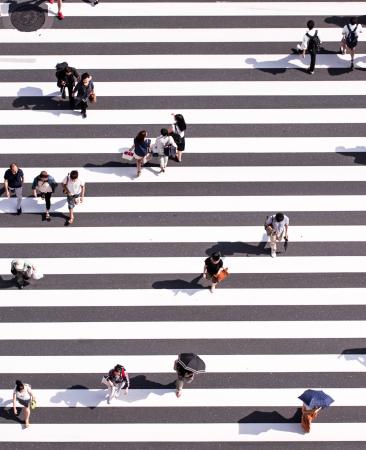
Introduction to Algorithmic Bias
Algorithms leverage existing data to predict an outcome, using inputs that are associated with the outcome. One problem with the forthcoming tide of machine learning algorithms is that such algorithms can be biased. In this blog, we start exploring the concept of "Algorithmic Bias"
Author: Caleb Hearn
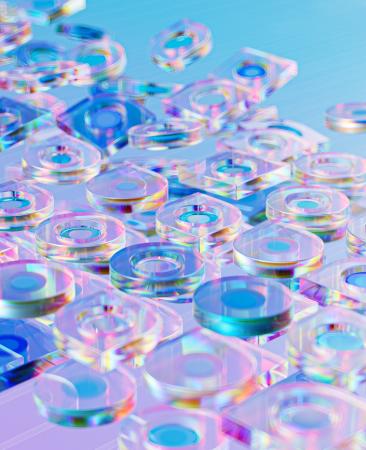
Introduction to Performance Drift
Algorithms are routinely used in the clinic to make decisions on patient care. Over time these algorithms may deteriorate in performance. Here, we start the explore the concept of “performance drift”.
Author: Likhitha Kolla
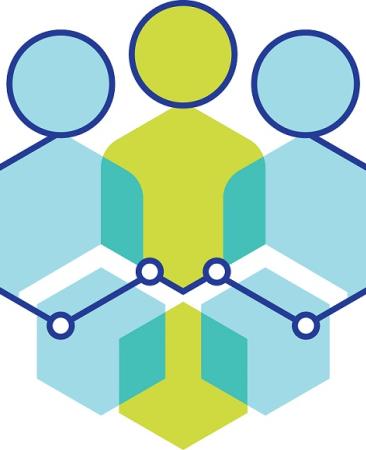
HACBlog: Staff Spotlight #1
Updates from the HACLab for August 2023
Author: Will Ferrell
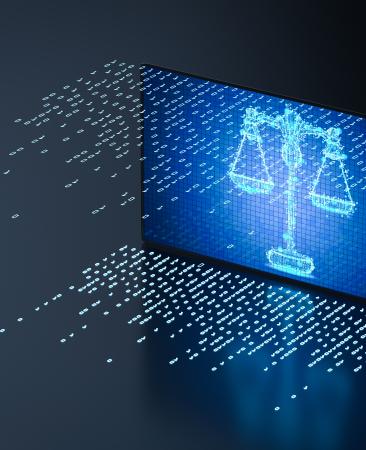
How do we regulate AI in healthcare?
AI's role in our daily lives continues to become more omnipresent and healthcare is no exception. As AI becomes more routinely incorporated in healthcare, a central question becomes – how do we regulate it?
Author: Ravi Parikh
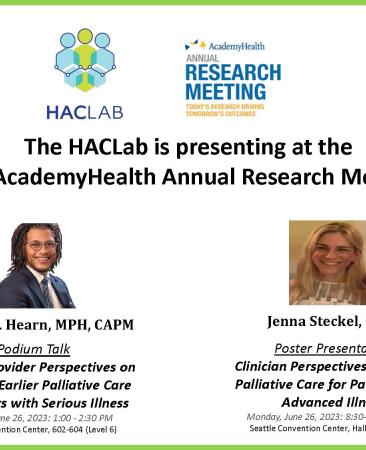
HACLab at the 2023 AcademyHealth Annual Research Meeting!
Caleb M. Hearn, MPH, CAPM will have a podium talk “Hospice Provider Perspectives on Providing Earlier Palliative Care for Patients with Serious Illness”
Jenna Steckel, MSW will have a poster presentation “Clinician Perspectives on Virtual Palliative Care for Patients with Advanced Illness”
What is..
Machine learning?
Cancer survivorship?
Algorithm unfairness?
Real World Data?
Patient Generated Health Data?
A branch of AI and computer science which focuses on the use of data and algorithms to imitate the way that humans learn, gradually improving its accuracy (source:IBM)
The health and well-being of a person with cancer from the time of diagnosis until the end of life. This includes issues related to follow-up care, late effects of treatment, cancer recurrence, second cancers, and quality of life (source:cancer.gov)
Systematic and repeatable errors in a computer system that create unfair outcomes, such as privileging one arbitrary group of users over others. It also, occurs when an algorithm produces results that are systemically prejudiced due to erroneous assumptions in the machine learning process (source:FSU)
The data relating to patient health status and/or the delivery of health care routinely collected from a variety of sources- electronic health records (EHRs), claims and billing activities, pghd, product and disease registries (source:FDA)
Health-related data created, recorded, or gathered by or from patients (or family members or other caregivers) to help address a health concern (source:healthit)