Introduction to Algorithmic Bias
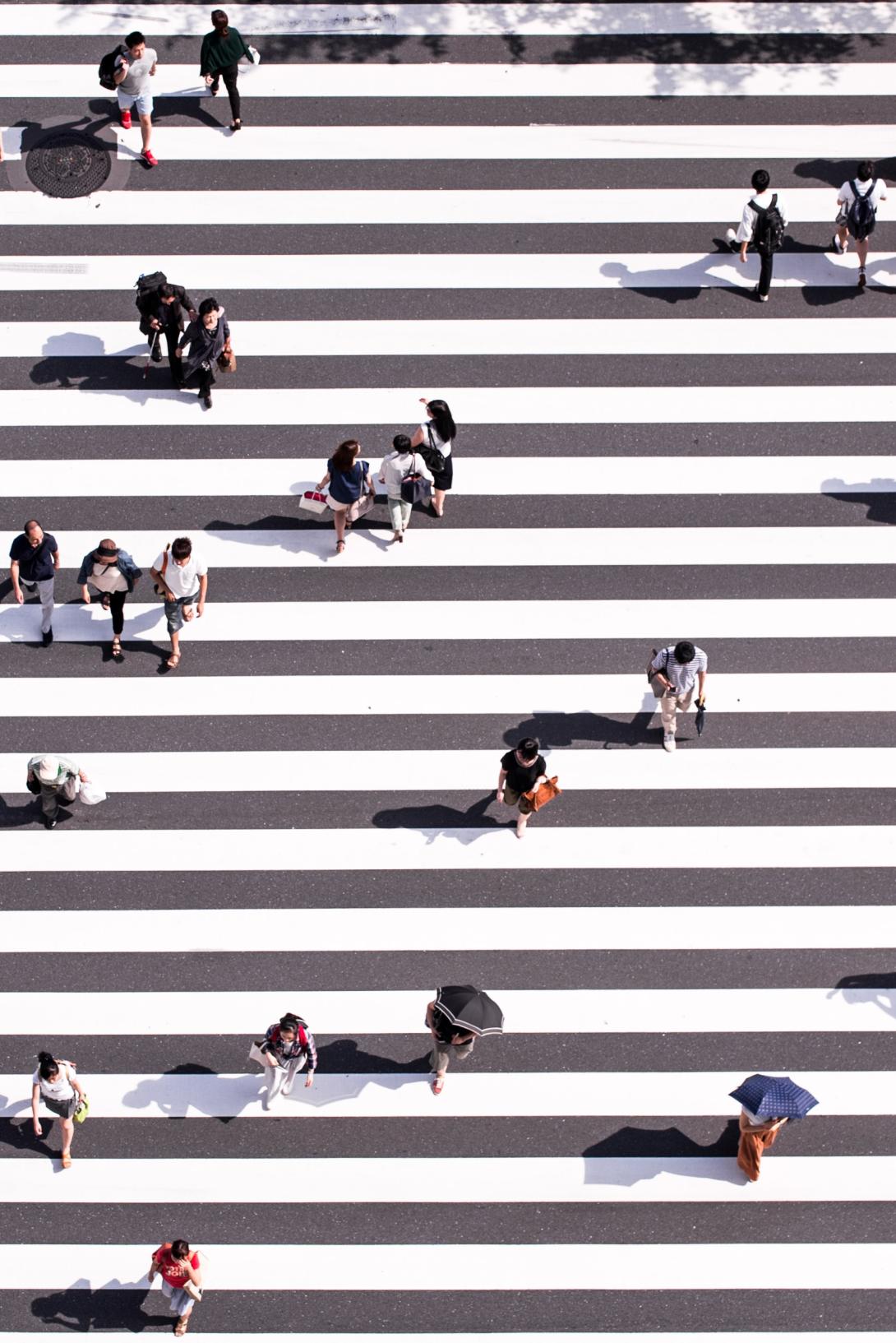
- Log in to post comments
Algorithms leverage existing data to predict an outcome, using inputs that are associated with the outcome. For instance, the Veterans’ Health Administration’s Care Assessment Needs (CAN) score utilizes clinical and administrative data collected during the routine course of a Veteran’s care to predict a patient’s individual risk of being hospitalized and/or dying in the forthcoming 3-12 months(1). The score is used to aid in care and resource allocation.
Algorithms have many practical applications in our society. For instance, the use of machine learning algorithms makes the interpretation of genetic data practical, aiding precision and genomic medical research and development.(2) The ability to utilize quantitative predictions using large sets can be leveraged in any setting that utilizes data.
One problem with the forthcoming tide of machine learning algorithms is that such algorithms can be biased. We look at bias as a systematic mischaracterization of risk in favor of or against a person or group of people (3). Bias can affect algorithms in many ways, but it isn’t often well-understood what contributes to bias.
Statistical bias is when the outcome doesn’t truly reflect the underlying true value. The algorithm predicts the wrong value or inaccurately represents reality due to various causes, including suboptimal sampling (4) or heterogeneity of treatment effects (5). One common cause of statistical bias is what we call the “outcome labels problem”. One example of the labels problem comes from Obermeyer et al’s Science study that examines an algorithm that uses healthcare spending as a proxy for health (6). They examine how this disadvantages Black populations, who have been systemically underserved by Medicine, by making them appear healthier. Ironically, this information could also be used to prove access inequities between races. We call this an “outcomes label problem” because healthcare spending is being used as a proxy for “health” which will disadvantage people who have historically spent less in this sector due to transgenerational harm and inequities of access. Another example is something called “heterogeneity of effects”. This is when the covariate-outcome relationship is different for different subgroups (like race, sex, treatment, etc.) (5). This was the case in the Framingham study where the association with heart attack and stroke where different by races (7,8). The lack of attention paid to the heterogeneity of effects in this 1976 study led to prevention programs for the general population to be built based on something that showed potential exclusively for white men.
Social bias is discrimination for, or against, a person or group, or a set of ideas or beliefs, in a way that is prejudicial or unfair (9). This is what most people are referring to when mentioning bias. Theoretically, you can apply different statistical methods or outcome labels within your own data to FIX statistical bias. Researchers and developers can't “fix” social bias in algorithms because they don't have the data necessary to. In healthcare algorithms, inequities and violence in care delivery and overrepresentation of white men in historical data cause the input and outcomes to reflect the bias of the data it's made of. Genetic testing is often used in breast cancer care to detect genetic markers of risk. Unfortunately, genetic testing is less likely to be performed for Black women (10,11). A group of individuals being less likely to receive genetic testing, weakens the ability of any program trained on these datasets to be able to pick up high-risk mutations within this population.
Algorithm bias is a byproduct of social and statistical biases of many forms. Many of these biases are areas of study within themselves that involve theories and concepts spanning from mathematics to social justice. From these concepts and theories, we can quantify how biased an algorithm is using various methods. There are emerging paradigms to monitor AI for bias that may emerge during its application. Ultimately, the million-dollar question is how to prevent algorithms of bias. We explore all these topics at the HACLab; stay tuned for future studies!
Caleb Hearn is a Senior Research Coordinator in the Department of Medical Ethics and Health Policy at the University of Pennsylvania.