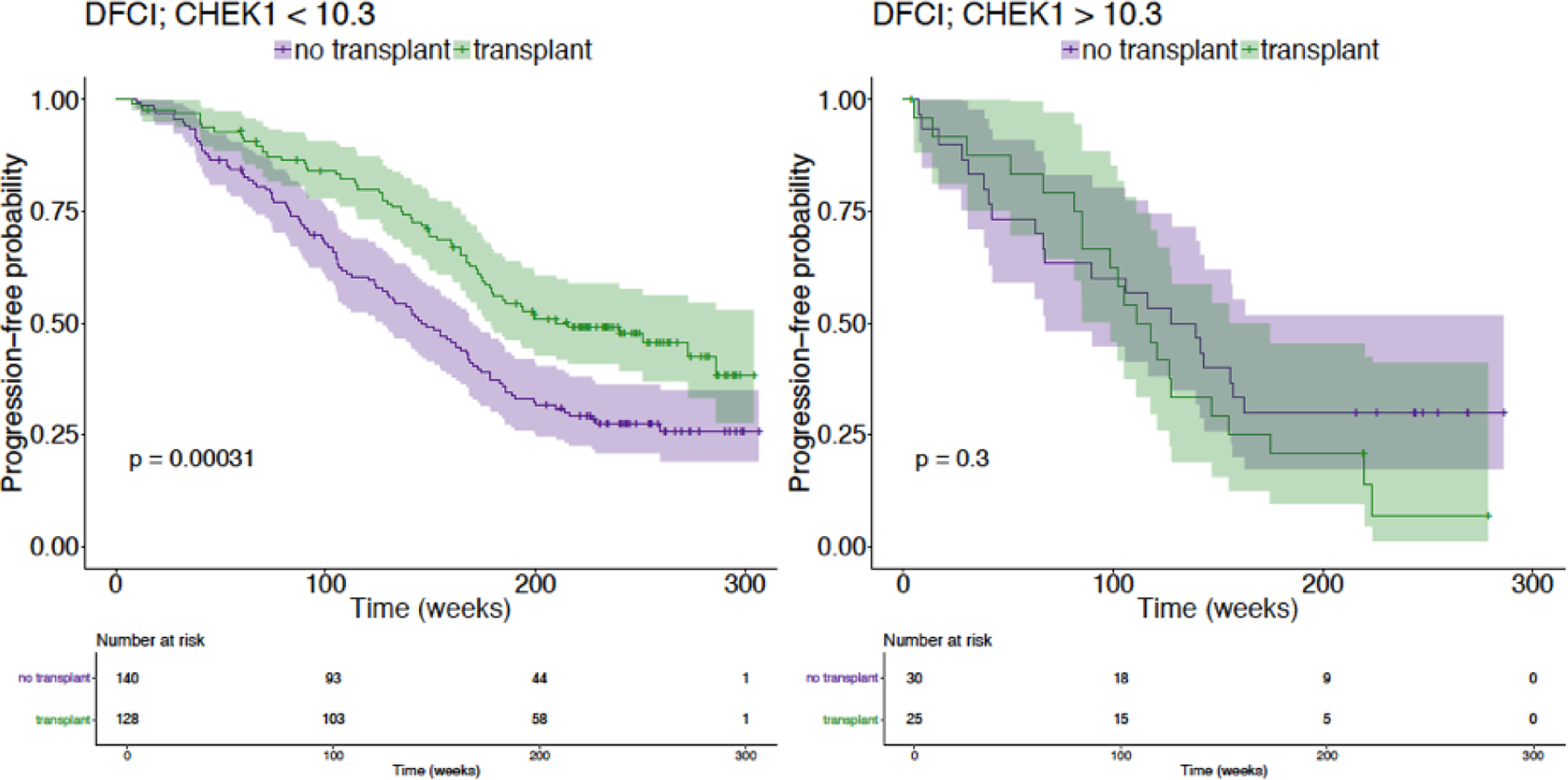
What is the problem
Clinical trials are the gold standard for approving oncology drugs. But only 10% of the US population will ever participate in a clinical trial, and trials are oftentimes expensive and take time to read out. How can we use cutting-edge techniques in causal inference and machine learning to simulate in-silico clinical trials for novel therapeutics and in underrepresented patient populations?
What are we doing
In one series of projects in collaboration with Flatiron Health, we are using machine learning algorithms to simulate in-silico trials of novel agents in breast, lung, and other cancers, to identify subpopulations that may not respond as favorably as cited in clinical trials. We have also simulated race-based differences in effectiveness of two common prostate cancer drugs.
Impact
There is a large debate about how observational data should be used for oncology drug approvals. We aim to produce high-quality evidence that establishes methodologic frameworks for in-silico trials. While we do not argue for replacing clinical trials, we believe that in-silico trials are a crucial adjunct to bring the most effective agents to phase III testing and to market.