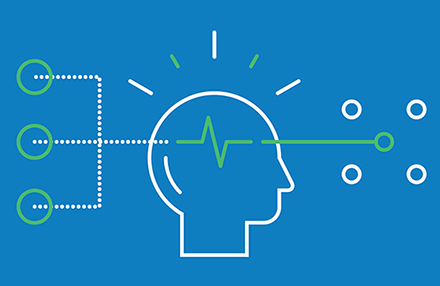
Per NIST standards, explainability refers to the representation of the mechanisms underlying AI systems’ operation and the meaning of AI systems’ output in the context of their designed functions. Our mixed-methods studies suggest that clinicians and health care workers are reluctant to use predictions that are made without explanations. For instance, in a qualitative study led by our group, oncologists who were exposed to a machine learning prognostic algorithm desired explanations behind those predictions before they would use the model in practice. In a unique collaboration between physicians and computer scientists, we are developing novel neurosymbolic methods to enforce explainations that clinicians can trust across diagnostic, prognostic, and treatment decision-making use cases. We have presented these methods at the ICML conference and have secured large-scale fundign to apply these ideas across many specialties and data modalities.