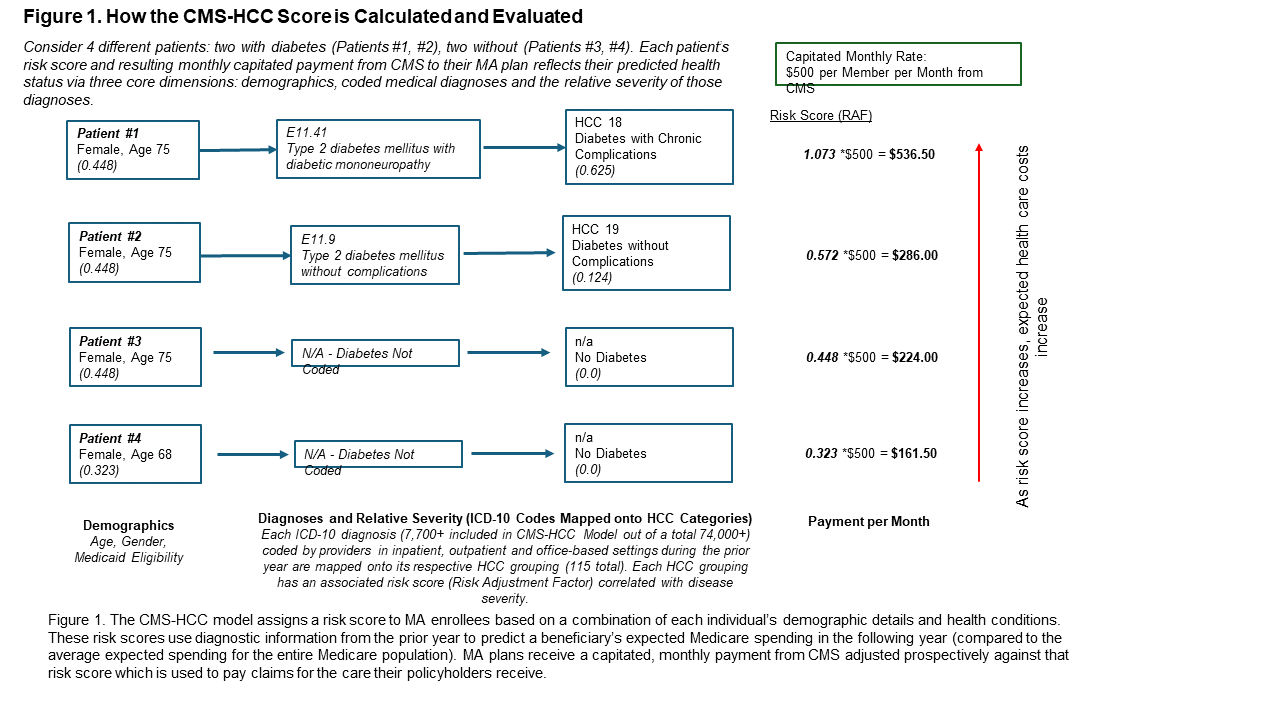
The federal government pays private Medicare Advantage health plans based on a risk adjustment methodology, Hierarchical Condition Categories, that relies on diagnosis codes and sociodemographic factors to predict expected costs of caring for each individual enrollee relative to the cost of the average Medicare beneficiary. With higher scores linked with higher payments, this approach has created perverse incentives among providers and payers, leading to significant overspending of taxpayer dollars. With the support of Schmidt Futures, we have convened a multi-institutional team of computer scientists, physicians and health policy researchers to improve upon the current HCC score. We are leveraging machine learning techniques to train and validate a more accurate and equitable HCC score with wide adoption potential, including testing adversarial ML techniques to counter upcoding. With MA enrollment growing rapidly, innovative solutions to improving risk adjustment will be a key part of the policy puzzle this next decade.