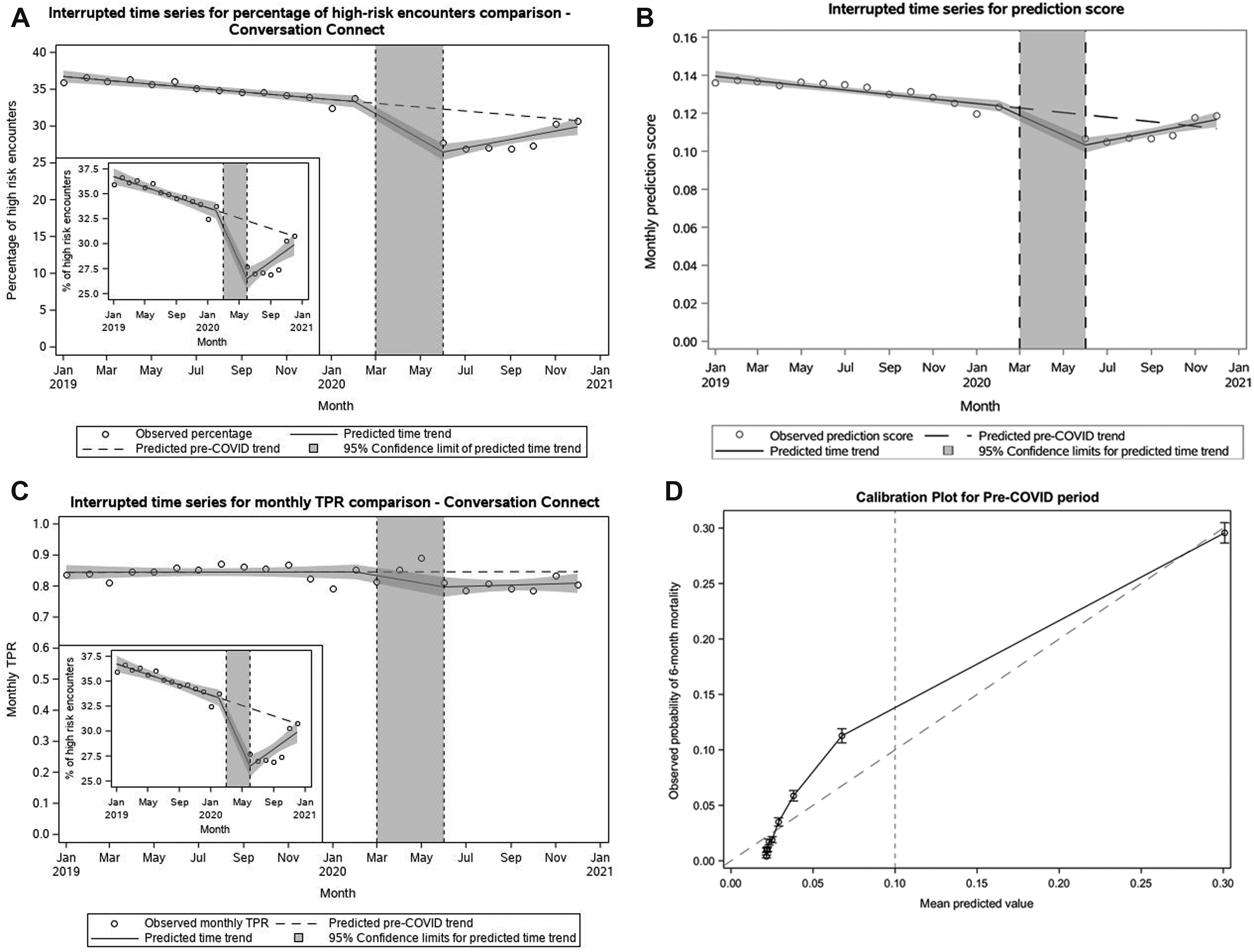
Predictive algorithms can help physicians identify high risk patients who need extra support. However, these algorithms decline in performance as healthcare practice patterns change with time. What drives this algorithmic performance drift and how can we modify these tools to combat it? The emergence of the COVID-19 pandemic introduced a sudden shock to healthcare operations. Our earlier study showed that declines in health lab utilization in 2020 was associated with performance drift in a mortality prediction algorithm, underreporting risk for advanced cancer patients. We are extending this work to an existing national risk algorithm to identify the extent and mechanisms of drift during the pandemic, impact on case management, and potential avenues to address future drift. We are applying lessons learned from this framework to other commercial and federal medical algorithms (i.e. VA CAN, CMS-HCC, Optum Impact Pro). Our work will inform strategies to detect ongoing drift in deployed models and improve reliability of predictive analytic tools, allowing for better risk-adjusted care.