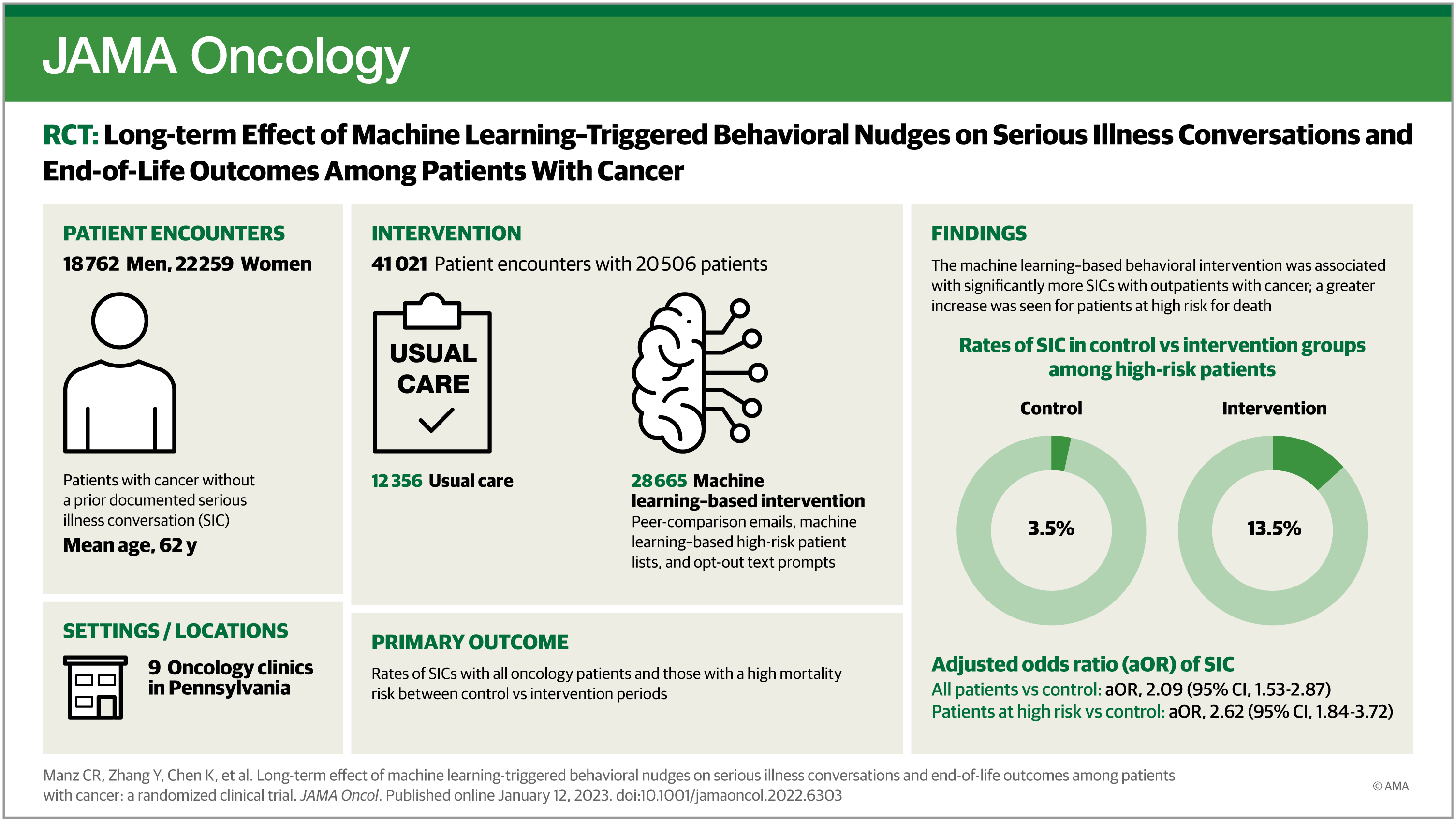
What is the problem
Clinicians have to balance making decisions around referrals, conversations, and treatment everyday. Algorithms offer a way to simplify this process, aiding clinicians in knowing when and for which patients bringing up tough conversations around such topics as palliative care would be appropriate. But little is known about how best to engage clinciains with these machine learning decision-aids. Using end of life care as a case study, we are examining how to present information to engender the most impact. How do we optimally integrate predicitive algorithms into clinicians' workflows?
What are we doing
Using mixed methods, we are learning providers' attitudes towards and likely behaviors when working with predictive algorithms. Through long form, semi-structured interviews, we asked clinicians and hospice executives and providers about referrals for people with serious advanced illness. These rigorous interviews allowed us to glean in-depth insights into programming, virtual care, communication, and referrals. In an NIH-funded study, we are using a nationwide survey to examine the effects of exposure to prognsostic estimates from machine learning algorithms and colleagues on thoracic oncologists' prognostic estimates and likelihood to engage patients in discussions around advanced care planning and palliative care.
Impact
With palliative care as a case study, we are learning from clinicians and hospice executives and providers about their practices and beliefs around referrals. This will help us design new comprehensive care models for a large insurer. In our NIH-funded work, we aim to improve how clinicians can work together with machine learning tools to improve prognostic accuracy, leading to more patients receiving palliative care.