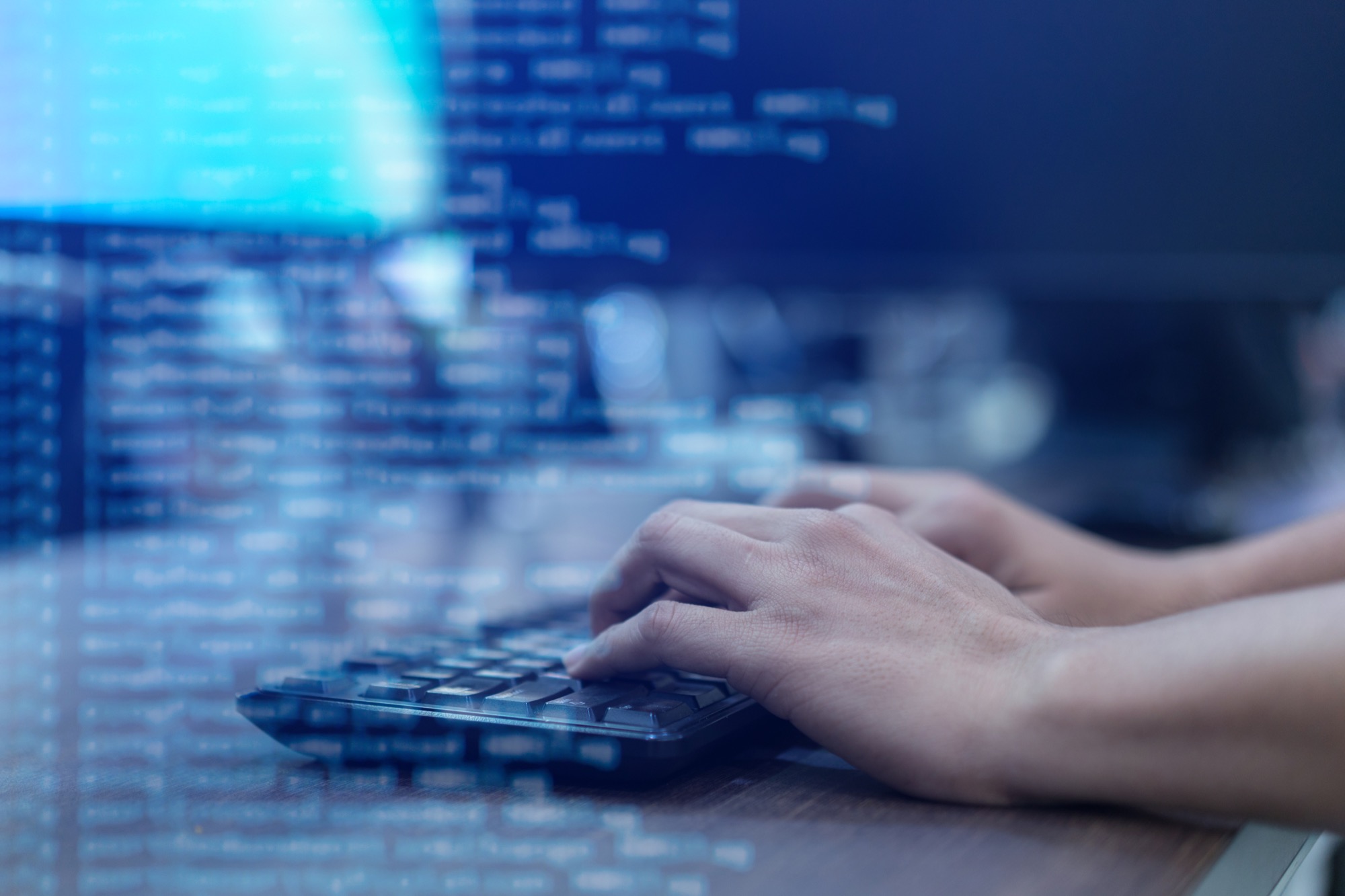
We train and validate models novel methodologies to improve detection and prognosis in healthcare. Specific topics of interest are 1) developing strategies to integrate sparse healthcare data into predictive models; 2) developing methods to detect and ameliorate performance drift in predictive models in real-time; and 3) developing explainable and trustworthy AI models. Our team collaborates with biostatistics and computer science faculty for much of this work.